Students Use Machine Learning in Lesson Designed to Reveal Issues, Promise of A.I.
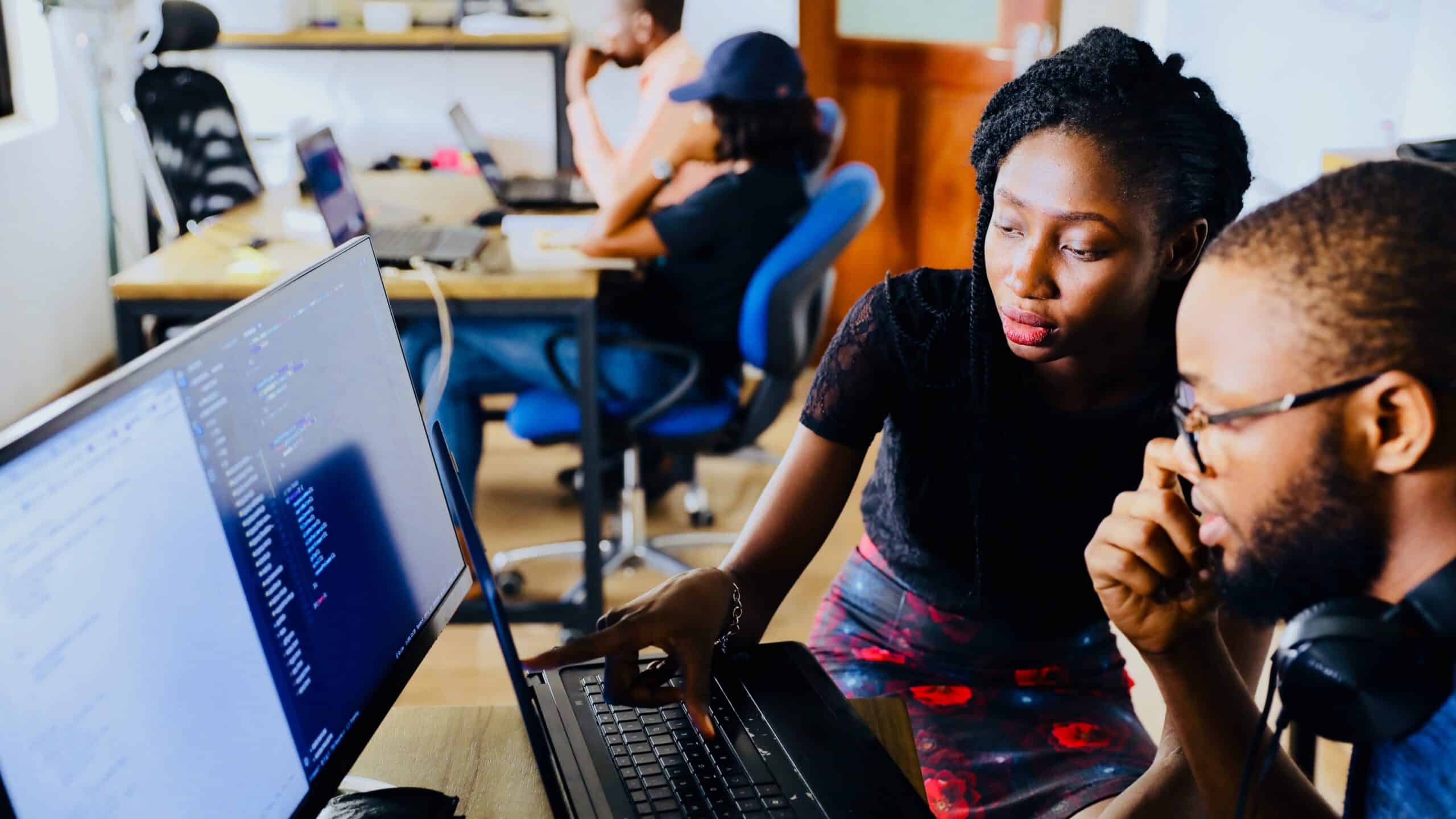
For Immediate Release
In a new study, North Carolina State University researchers had 28 high school students create their own machine-learning artificial intelligence (AI) models for analyzing data. The goals of the project were to help students explore the challenges, limitations and promise of AI, and to ensure a future workforce is prepared to make use of AI tools.
The study was conducted in conjunction with a high school journalism class in the Northeast. Since then, researchers have expanded the program to high school classrooms in multiple states, including North Carolina. NC State researchers are looking to partner with additional schools to collaborate in bringing the curriculum into classrooms.
“We want students, from a very young age, to open up that black box so they aren’t afraid of AI,” said the study’s lead author Shiyan Jiang, assistant professor of learning design and technology at NC State. “We want students to know the potential and challenges of AI, and so they think about how they, the next generation, can respond to the evolving role of AI and society. We want to prepare students for the future workforce.”
For the study, researchers developed a computer program called StoryQ that allows students to build their own machine-learning models. Then, researchers hosted a teacher workshop about the machine learning curriculum and technology in one-and-a-half hour sessions each week for a month. For teachers who signed up to participate further, researchers did another recap of the curriculum for participating teachers, and worked out logistics.
“We created the StoryQ technology to allow students in high school or undergraduate classrooms to build what we call ‘text classification’ models,” Jiang said. “We wanted to lower the barriers so students can really know what’s going on in machine-learning, instead of struggling with the coding. So we created StoryQ, a tool that allows students to understand the nuances in building machine-learning and text classification models.”
A teacher who decided to participate led a journalism class through a 15-day lesson where they used StoryQ to evaluate a series of Yelp reviews about ice cream stores. Students developed models to predict if reviews were “positive” or “negative” based on the language.
“The teacher saw the relevance of the program to journalism,” Jiang said. “This was a very diverse class with many students who are under-represented in STEM and in computing. Overall, we found students enjoyed the lessons a lot, and had great discussions about the use and mechanism of machine-learning.”
Researchers saw that students made hypotheses about specific words in the Yelp reviews, which they thought would predict if a review would be positive, or negative. For example, they expected reviews containing the word “like” to be positive. Then, the teacher guided the students to analyze whether their models correctly classified reviews. For example, a student who used the word “like” to predict reviews found that more than half of reviews containing the word were actually negative. Then, researchers said students used trial and error to try to improve the accuracy of their models.
“Students learned how these models make decisions, and the role that humans can play in creating these technologies, and the kind of perspectives that can be brought in when they create AI technology,” Jiang said.
From their discussions, researchers found that students had mixed reactions to AI technologies. Students were deeply concerned, for example, about the potential to use AI to automate processes for selecting students or candidates for opportunities like scholarships or programs.
For future classes, researchers created a shorter, five-hour program. They’ve launched the program in two high schools in North Carolina, as well as schools in Georgia, Maryland and Massachusetts. In the next phase of their research, they are looking to study how teachers across disciplines collaborate to launch an AI-focused program and create a community of AI learning.
“We want to expand the implementation in North Carolina,” Jiang said. “If there are any schools interested, we are always ready to bring this program to a school. Since we know teachers are super busy, we’re offering a shorter professional development course, and we also provide a stipend for teachers. We will go into the classroom to teach if needed, or demonstrate how we would teach the curriculum so teachers can replicate, adapt, and revise it. We will support teachers in all the ways we can.”
The study, “High school students’ data modeling practices and processes: From modeling unstructured data to evaluating automated decisions,” was published online March 13 in the journal Learning, Media and Technology. Co-authors included Hengtao Tang, Cansu Tatar, Carolyn P. Rosé and Jie Chao. The work was supported by the National Science Foundation under grant number 1949110.
-oleniacz-
Note to Editors: The study abstract follows.
“High school students’ data modeling practices and processes: From modeling unstructured data to evaluating automated decisions”
Authors: Shiyan Jiang, Hengtao Tang, Cansu Tatar, Carolyn P. Rosé and Jie Chao.
Published: March 13, 2023, Learning, Media and Technology
DOI: 10.1080/17439884.2023.2189735
Abstract: It’s critical to foster artificial intelligence (AI) literacy for high school students, the first generation to grow up surrounded by AI, to understand working mechanism of data-driven AI technologies and critically evaluate automated decisions from predictive models. While efforts have been made to engage youth in understanding AI through developing machine learning models, few provided in-depth insights into the nuanced learning processes. In this study, we examined high school students’ data modeling practices and processes. Twenty-eight students developed machine learning models with text data for classifying negative and positive reviews of ice cream stores. We identified nine data modeling practices that describe students’ processes of model exploration, development, and testing and two themes about evaluating automated decisions from data technologies. The results provide implications for designing accessible data modeling experiences for students to understand data justice as well as the role and responsibility of data modelers in creating AI technologies.
- Categories: