New Data Processing Module Makes Deep Neural Networks Smarter
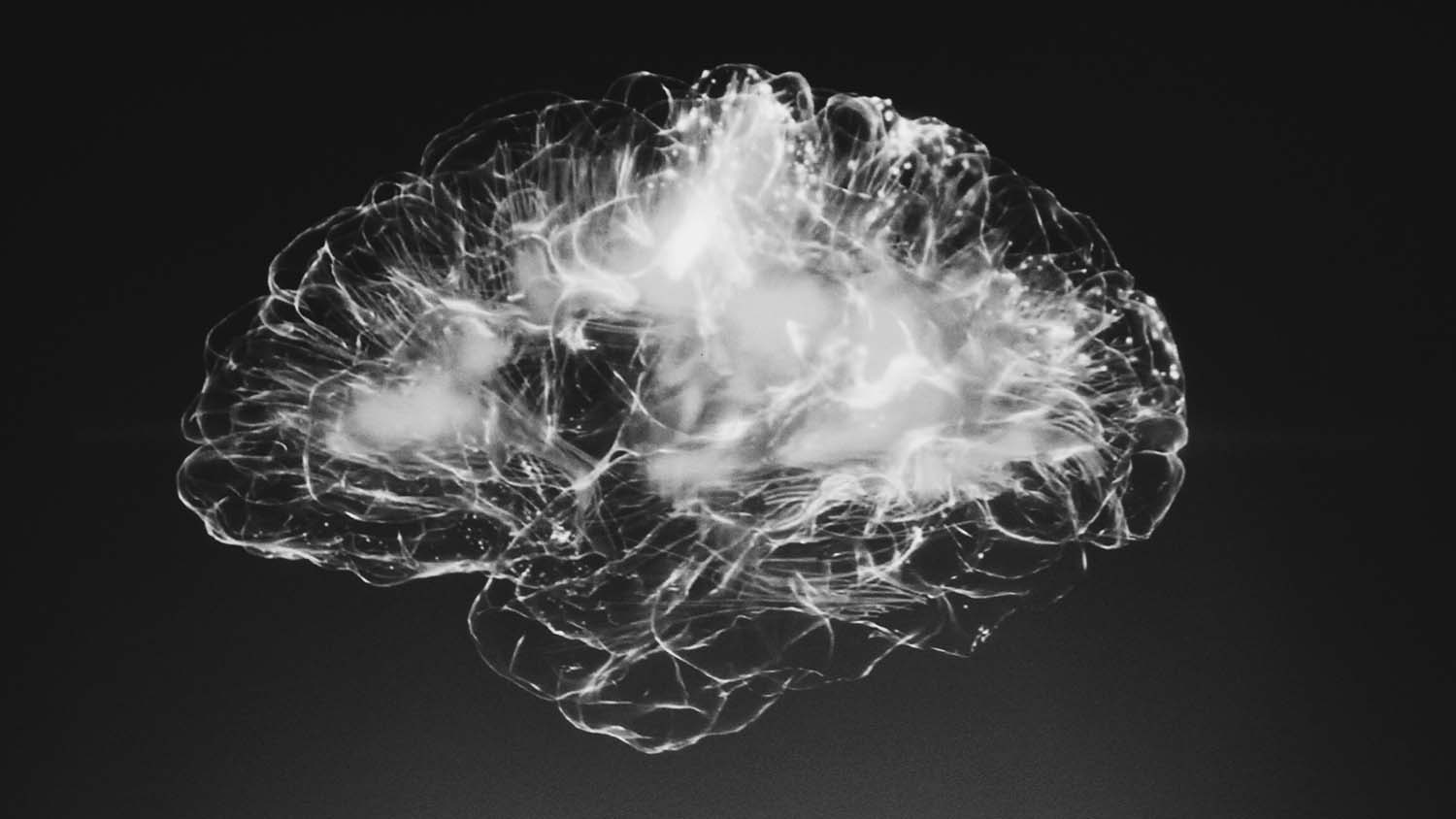
For Immediate Release
Artificial intelligence researchers at North Carolina State University have improved the performance of deep neural networks by combining feature normalization and feature attention modules into a single module that they call attentive normalization (AN). The hybrid module improves the accuracy of the system significantly, while using negligible extra computational power.
“Feature normalization is a crucial element of training deep neural networks, and feature attention is equally important for helping networks highlight which features learned from raw data are most important for accomplishing a given task,” says Tianfu Wu, corresponding author of a paper on the work and an assistant professor of electrical and computer engineering at NC State. “But they have mostly been treated separately. We found that combining them made them more efficient and effective.”
To test their AN module, the researchers plugged it into four of the most widely used neural network architectures: ResNets, DenseNets, MobileNetsV2 and AOGNets. They then tested the networks against two industry standard benchmarks: the ImageNet-1000 classification benchmark and the MS-COCO 2017 object detection and instance segmentation benchmark.
“We found that AN improved performance for all four architectures on both benchmarks,” Wu says. “For example, top-1 accuracy in the ImageNet-1000 improved by between 0.5% and 2.7%. And Average Precision (AP) accuracy increased by up to 1.8% for bounding box and 2.2% for semantic mask in MS-COCO.
“Another advantage of AN is that it facilitates better transfer learning between different domains,” Wu says. “For example, from image classification in ImageNet to object detection and semantic segmentation in MS-COCO. This is illustrated by the performance improvement in the MS-COCO benchmark, which was obtained by fine-tuning ImageNet-pretrained deep neural networks in MS-COCO, a common workflow in state-of-the-art computer vision.
“We have released the source code and hope our AN will lead to better integrative design of deep neural networks.”
The paper, “Attentive Normalization,” was presented at the European Conference on Computer Vision (ECCV), which was held online Aug. 23-28. The paper was co-authored by Xilai Li, a recent Ph.D. graduate from NC State; and by Wei Sun, a Ph.D. student at NC State. The work was done with support from the National Science Foundation, under grants 1909644, 1822477, and 2013451; and by the U.S. Army Research Office, under grant W911NF1810295.
-shipman-
Note to Editors: The study abstract follows.
“Attentive Normalization”
Authors: Xilai Li, Wei Sun, and Tianfu Wu, North Carolina State University
Presented: 16th European Conference on Computer Vision, held online Aug. 23-28
Abstract: In state-of-the-art deep neural networks, both feature normalization and feature attention have become ubiquitous. They are usually studied as separate modules, however. In this paper, we propose a light-weight integration between the two schema and present Attentive Normalization (AN). Instead of learning a single affine transformation, AN learns a mixture of affine transformations and utilizes their weighted sum as the final affine transformation applied to re-calibrate features in an instance-specific way. The weights are learned by leveraging channel-wise feature attention. In experiments, we test the proposed AN using four representative neural architectures in the ImageNet-1000 classification benchmark and the MS-COCO 2017 object detection and instance segmentation benchmark. AN obtains consistent performance improvement for different neural architectures in both benchmarks with absolute increase of top-1 accuracy in ImageNet-1000 between 0.5% and 2.7%, and absolute increase up to 1.8% and 2.2% for bounding box and mask AP in MS-COCO respectively. We observe that the proposed AN provides a strong alternative to the widely used Squeeze-and-Excitation (SE) module. The source codes are publicly available at the ImageNet Classification Repo (https://github.com/iVMCL/AOGNet-v2) and the MS-COCO Detection and Segmentation Repo (https://github.com/iVMCL/AttentiveNorm_Detection).