Researchers Can Trace Dust Samples Using Fungal DNA
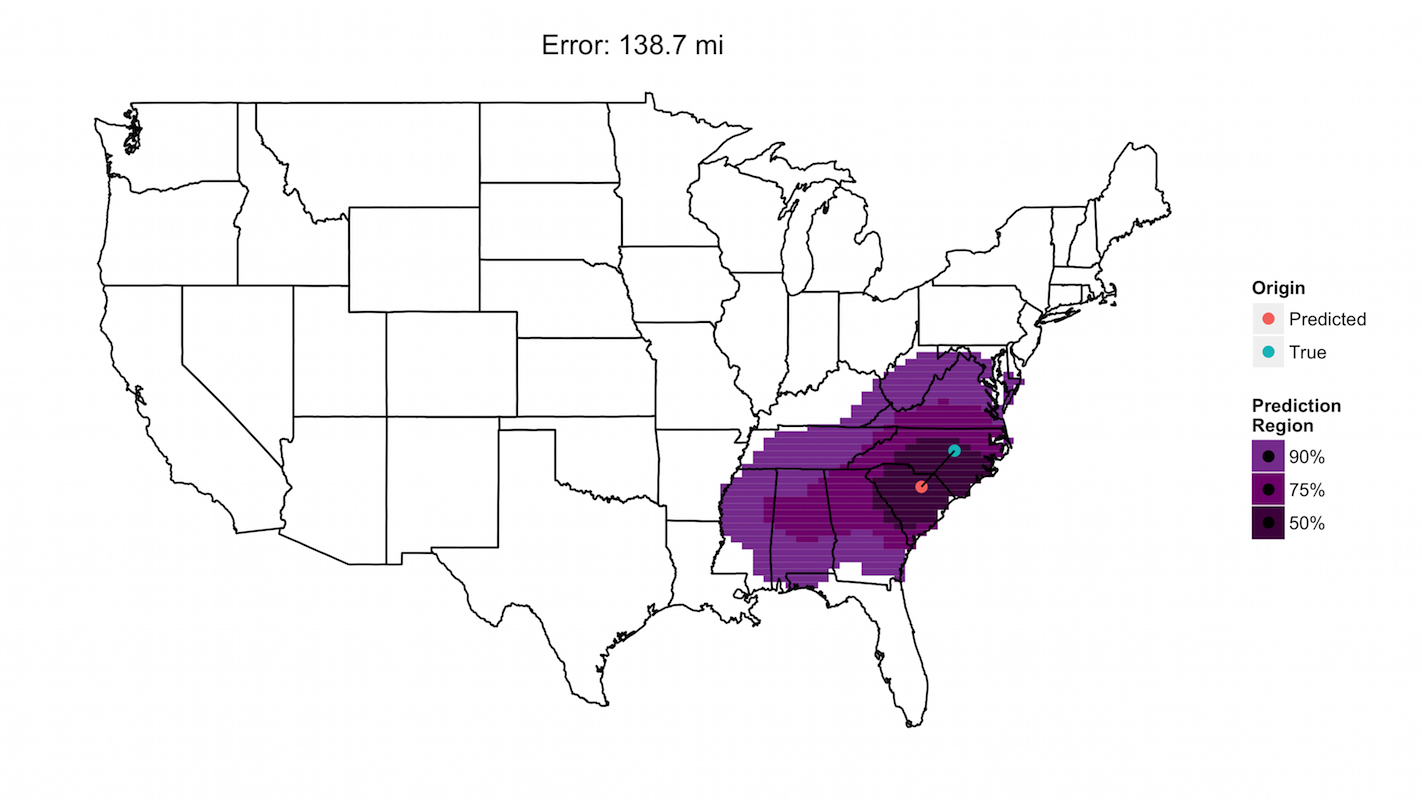
For Immediate Release
Researchers from North Carolina State University and the University of Colorado, Boulder, have developed a statistical model that allows them to tell where a dust sample came from within the continental United States based on the DNA of fungi found in the sample.
The primary goal of the research was to develop a new forensic biology tool for law enforcement or archaeologists. “But it may also give us a greater understanding of the invisible ecosystems of microbial life that we know are all around us, but that we don’t fully comprehend,” says Neal Grantham, a Ph.D. student in statistics at NC State and lead author of a paper on the work.
The researchers developed the model using data from the Wild Life of Our Homes citizen science project conducted by the Your Wild Life lab based at NC State. The project collected dust samples from approximately 1,000 homes across the continental U.S., including samples from 47 of the 48 contiguous states.
The goal of that project was to test the dust samples for DNA to identify the microbial species present in and around our homes. One of the things the project found was that the types of fungus – or fungal taxa – varied widely from region to region.
“Based on that finding, we wanted to determine if you could predict where a dust sample came from based on the fungi present in the sample, and – most of the time – we can,” Grantham says.
The researchers developed a model that analyzed the fungal taxa present in a dust sample and predicted where the sample came from. About 5 percent of the time, the model’s predictions were within 35 miles of the correct sampling site. Those were the most accurate predictions. The worst 5 percent were off by at least 645 miles. The model’s median prediction error was 143 miles. However, the research team is already working to make the model more accurate by developing more advanced algorithms.
“The work we’ve done so far was to determine whether this concept was viable,” Grantham says. “Now that we know it is viable, we’re developing statistical methods that are better suited to the problem.
“Ultimately, we want to have an online tool for law enforcement to run the results of dust samples taken from a piece of clothing, a body, or a vehicle, and get information on where the clothing, body, or vehicle has been,” Grantham says.
The paper, “Fungi Identify the Geographic Origin of Dust Samples,” was published online in the journal PLOS ONE on April 13, 2015. The paper was co-authored by Brian Reich, Krishna Pacifici, Eric Laber, Holly Menninger and Rob Dunn of NC State; and by Jessica Henley, Albert Barberán, Jonathan Leff and Noah Fierer of CU-Boulder. The work was supported by the Sloan Foundation.
-shipman-
Note to Editors: The study abstract follows.
“Fungi Identify the Geographic Origin of Dust Samples”
Authors: Neal Grantham, Brian Reich, Krishna Pacifici, Eric Laber, Holly Menninger and Rob Dunn, North Carolina State University; Jessica Henley, Albert Barberán, Jonathan Leff and Noah Fierer, University of Colorado, Boulder
Published: April 13, PLOS ONE
DOI: 10.1371/journal.pone.0122605
Abstract: There is a long history of archaeologists and forensic scientists using pollen found in a dust sample to identify its geographic origin or history. Such palynological approaches have important limitations as they require time-consuming identification of pollen grains, a priori knowledge of plant species distributions, and a sufficient diversity of pollen types to permit spatial or temporal identification. We demonstrate an alternative approach based on DNA sequencing analyses of the fungal diversity found in dust samples. Using nearly 1,000 dust samples collected from across the continental U.S., our analyses identify up to 40,000 fungal taxa from these samples, many of which exhibit a high degree of geographic endemism. We develop a statistical learning algorithm via discriminant analysis that exploits this geographic endemicity in the fungal diversity to correctly identify samples to within a few hundred kilometers of their geographic origin with high probability. In addition, our statistical approach provides a measure of certainty for each prediction, in contrast with current palynology methods that are almost always based on expert opinion and devoid of statistical inference. Fungal taxa found in dust samples can therefore be used to identify the origin of that dust and, more importantly, we can quantify our degree of certainty that a sample originated in a particular place. This work opens up a new approach to forensic biology that could be used by scientists to identify the origin of dust or soil samples found on objects, clothing, or archaeological artifacts.
- Categories: