New Tool Tackles Uncertainty in Military Logistics Planning
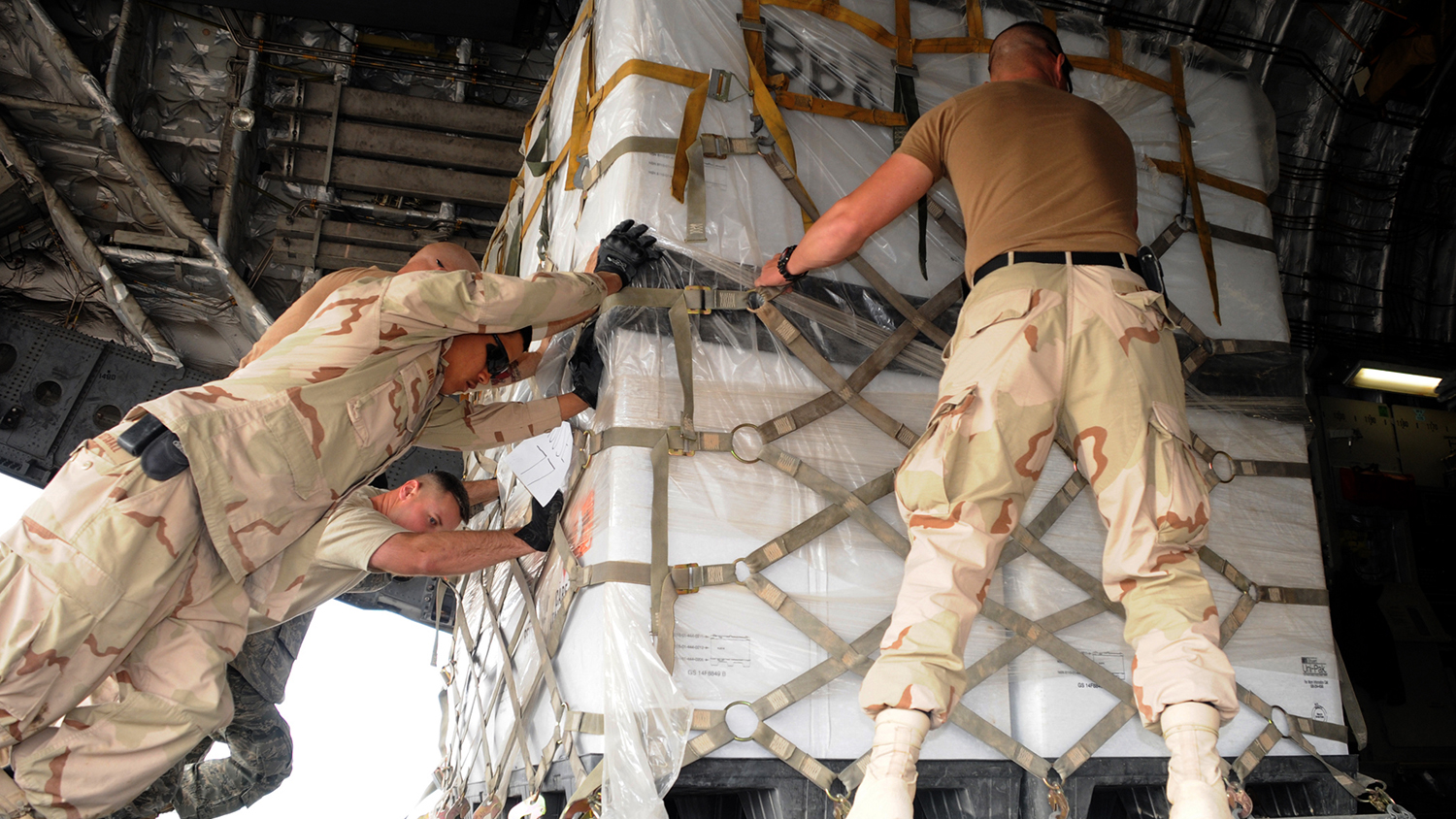
For Immediate Release
Military deployments to austere environments – whether humanitarian missions or combat operations – involve extensive logistical planning, which is often complicated by unforeseen events. Researchers at North Carolina State University have created a model to help military leaders better account for logistical risk and uncertainty during operational planning and execution.
“Every branch of the military now uses an enterprise resource planning (ERP) system that handles everything from requisitions to shipment of supplies to inventory tracking,” says Brandon McConnell, corresponding author of a paper on the new model and a research assistant professor in NC State’s Edward P. Fitts Department of Industrial and Systems Engineering. “These ERP systems make it possible to create computational models that can be used to identify the most efficient means of meeting the military’s logistical needs.
“These models would be particularly valuable during expeditionary operations, in which the military is seeking to establish its presence – and its supply chain – in an environment that is subject to a fair amount of uncertainty.
“The model that we’ve developed can not only facilitate the military’s ability to efficiently determine what will be needed where, but can also assess risk in near real time in order to account for uncertainty,” says McConnell, a former infantry captain in the U.S. Army who served two tours in Iraq.
The new model, called the Military Logistics Network Planning System (MLNPS), draws on three sources of information. First is logistical data from the ERP system. Second is operational data, such as an operation’s mission, organization and timeline. Third is data on “mission specific demand,” meaning logistical requirements that are particular to a given mission and its environment. For example, a combat operation being conducted in a cold, damp environment would have different requirements than a humanitarian mission being conducted in a hot, dry environment.
The MLNPS also uses two factors to assess risk and determine how risk might affect military capacities. The first factor is the likelihood that an event will happen; the second factor is what the consequences of that event will be. For example, if the likelihood of two events is identical, the model would give more weight to the event that could have a greater adverse impact on military personnel and mission performance.
“The MLNPS uses all of the available data, accounts for risk, then forecasts what the logistical outcomes will look like in reality,” McConnell says. “The MLNPS can be used as a decision planning aid, allowing leaders to test-drive plans in order to identify courses of action that will best support carrying out an operation.”
The MLNPS could also be used while an operation is being executed, as part of contingent logistical planning efforts that take place as circumstances change on the ground.
“Right now, the MLNPS is a robust proof-of-concept prototype, designed to demonstrate the potential value of powerful computational tools that can make use of ERP systems,” McConnell says. “Existing logistical tools are both valuable and powerful. However, I’m not aware of any other methods that make use of ERP data and are also fast enough for operational use when time is of the essence.”
“This research lays the mathematical and operational foundation for construction of a network-based model that captures routing alternatives and characterizes solutions for capacity planning and resiliency analysis in near-real time,” says Joseph Myers, Army Research Office mathematical sciences division chief at the Combat Capabilities Development Command’s Army Research Laboratory. “This project will provide military logistics planners with capabilities that are currently lacking in prevalent logistics planning tools.”
The paper, “Assessing Uncertainty and Risk in an Expeditionary Military Logistics Network,” is published in the Journal of Defense Modeling & Simulation. The paper was co-authored by Thom Hodgson and James Wilson, professors emeritus in the Fitts Department of Industrial and Systems Engineering; Michael Kay and Yunan Liu, associate professors in the Fitts Department; Russell King, the Henry Armfield Foscue Distinguished Professor in the Fitts Department; Greg H. Parlier, adjunct professor in the Fitts Department; and Kristin Thoney-Barletta, associate professor of textile and apparel, technology and management in NC State’s Wilson College of Textiles. The authors earned the 2019 Barchi Prize for best paper from the Military Operations Research Society.
Support for this research was provided by the Army Research Office under grant number W911NF1910055.
-shipman-
Note to Editors: The study abstract follows.
“Assessing Uncertainty and Risk in an Expeditionary Military Logistics Network”
Authors: Brandon M. McConnell, Thom J. Hodgson, Michael G. Kay, Russell E. King, Yunan Liu, Greg H. Parlier, Kristin Thoney-Barletta and James R. Wilson, North Carolina State University
Published: July 10, Journal of Defense Modeling & Simulation
DOI: 10.1177/1548512919860595
Abstract: Uncertainty is rampant in military expeditionary operations spanning high intensity combat to humanitarian operations. These missions require rapid planning and decision-support tools to address the logistical challenges involved in providing support in often austere environments. The U.S. Army’s adoption of an enterprise resource planning (ERP) system provides an opportunity to develop automated decision-support tools and other analytical models designed to take advantage of newly available logistical data. This research presents a tool that runs in near-real time to assess risk while conducting capacity planning and performance analysis designed for inclusion in a suite of applications dubbed the Military Logistics Network Planning System (MLNPS) which previously only evaluated the mean sample path. Logistical data from combat operations during Operation Iraqi Freedom (OIF) drives supply requisition forecasts for a contingency scenario in a similar geographic environment. A nonstationary queueing network model is linked with a heuristic logistics scheduling methodology to provide a stochastic framework to account for uncertainty and assess risk.