Model Aims to Help First Responders Reach Accident Sites Faster
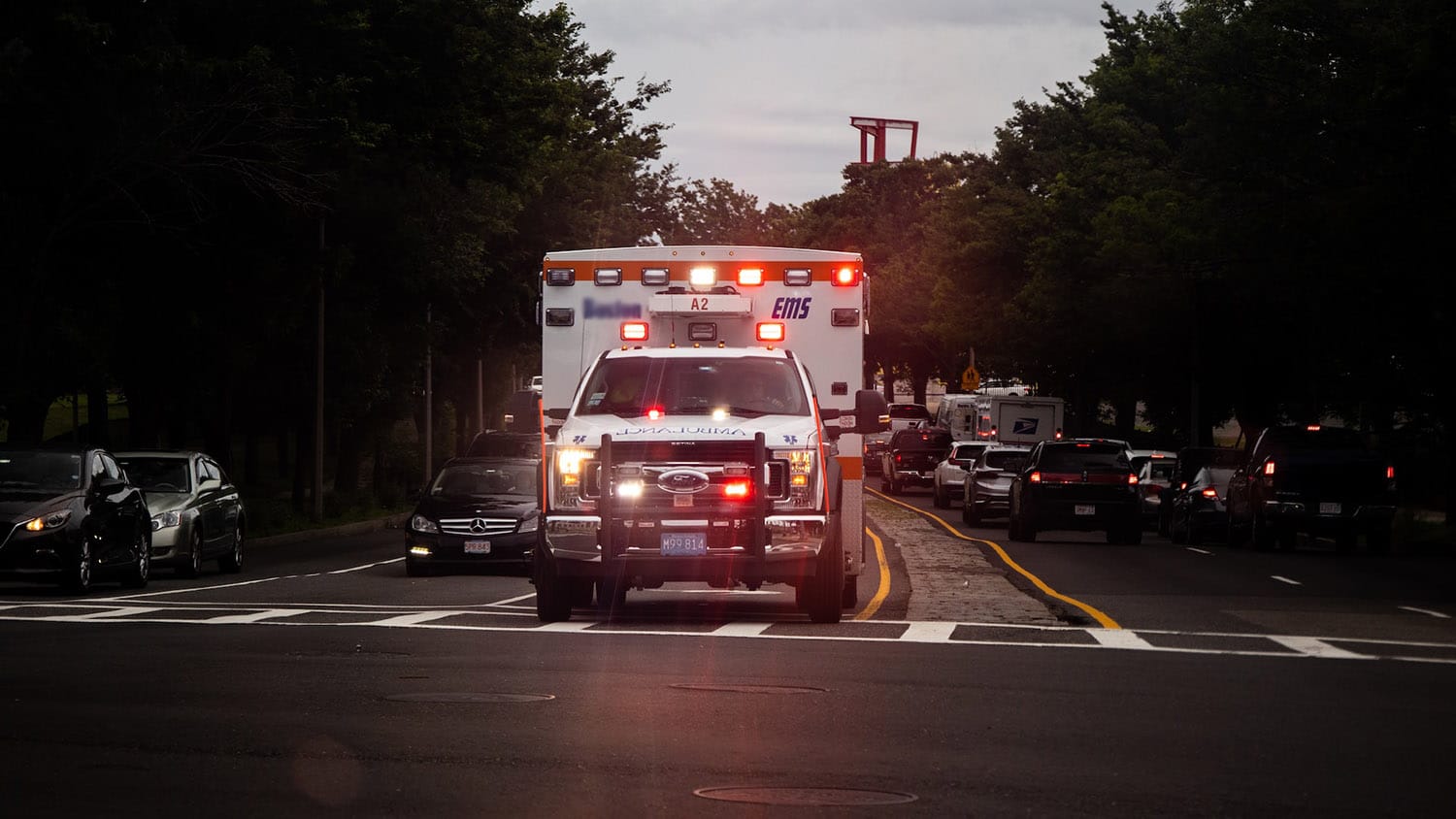
For Immediate Release
Researchers at North Carolina State University have developed a complex model to improve how quickly first responders – such as police and EMTs – reach the scene of vehicle accidents. In computational testing, the model outperformed the existing techniques for getting first responders to accident sites quickly.
“The goal was to figure out the most efficient way to get first responders to an accident,” says Leila Hajibabai, corresponding author of a paper on the work and an assistant professor in NC State’s Edward P. Fitts Department of Industrial and Systems Engineering. “Where should first responders be based in order to respond to the most likely sites of accidents? Is it always best for the closest first responders to go to an accident site, or – depending on traffic – could it be faster for first responders who are farther away to respond? How does accident severity affect response times? These are some of the questions our model addresses.”
To that end, the researchers developed a model that both maximizes the coverage area, so response units can respond to as many possible accident sites as possible, and minimizes the amount of time it would take respondents to reach accident sites. The model also accounts for “hot spots,” prioritizing the efficiency of response times to locations where accidents are most likely to happen based on historical data.
“The model can be used for both long-term planning and for allocating incident response resources on a day-to-day basis,” Hajibabai says.
For long-term planning, the model can help authorities optimize the location of respondents – i.e., help them determine where to locate first-responder infrastructure. On a day-to-day basis, the model could also help authorities determine which respondents are best placed to reach an accident most quickly.
To test the model, the researchers drew on data collected by the North Carolina Department of Transportation regarding 10,983 traffic incidents that occurred in 10,672 different locations in Raleigh, N.C. The researchers used the data to test how efficiently the model performed as compared to the two current state-of-the-art techniques used to allocate incident response resources.
“Our model outperformed the existing models in terms of improving response times, regardless of the size of the traffic problem they were responding to,” Hajibabai says.
What’s more, the researchers made the model sufficiently flexible to incorporate changes in the amount of resources available for incident response.
“Budgets and other circumstances can change significantly over time, and it’s important for our model to be able to incorporate changes in how many respondents are available,” Hajibabai says.
The researchers are now testing the limits of the model.
“Are there things we can do to make the model even faster? This is a proof-of-concept paper, and the results are excellent,” Hajibabai says. “Now we want to see what we can do to make it even better.” The researchers are looking for partners to conduct pilot studies with their model.
The paper, “Joint Column Generation and Lagrangian Relaxation Technique for Incident Respondent Location and Allocation,” is published open access in the journal Computer-Aided Civil and Infrastructure Engineering. First author of the paper is Asya Atik, a Ph.D. student at NC State.
-shipman-
Note to Editors: The study abstract follows.
“Joint Column Generation and Lagrangian Relaxation Technique for Incident Respondent Location and Allocation”
Authors: Asya Atik and Leila Hajibabai, North Carolina State University
Published: May 4, Computer-Aided Civil and Infrastructure Engineering
DOI: 10.1111/mice.13016
Abstract: Incident response operations require effective planning of resources to ensure timely clearance of roadways and avoidance of secondary incidents. This study formulates a mixed-integer linear program to minimize the total expected travel time and maximize the demand covered. The model accounts for the location, severity, frequency of incidents, dispatching locations, and availability of incident respondents. An integrated methodology that includes column generation and Lagrangian relaxation with a density-based clustering technique that defines incident hot-spots is proposed. The hybrid approach is applied to an empirical case study in Raleigh, NC. A network instance with 10,672 incident sites, clustered with a search distance (ε) of 5 min, is solved efficiently with an optimality gap of 1.37% in 2 min. A Benders decomposition technique is implemented to conduct benchmark analyses. The numerical results suggest that the proposed algorithm can solve the problem efficiently and outperform the benchmark solutions.