Study: U.S. Flood Damage Risk Is Underestimated
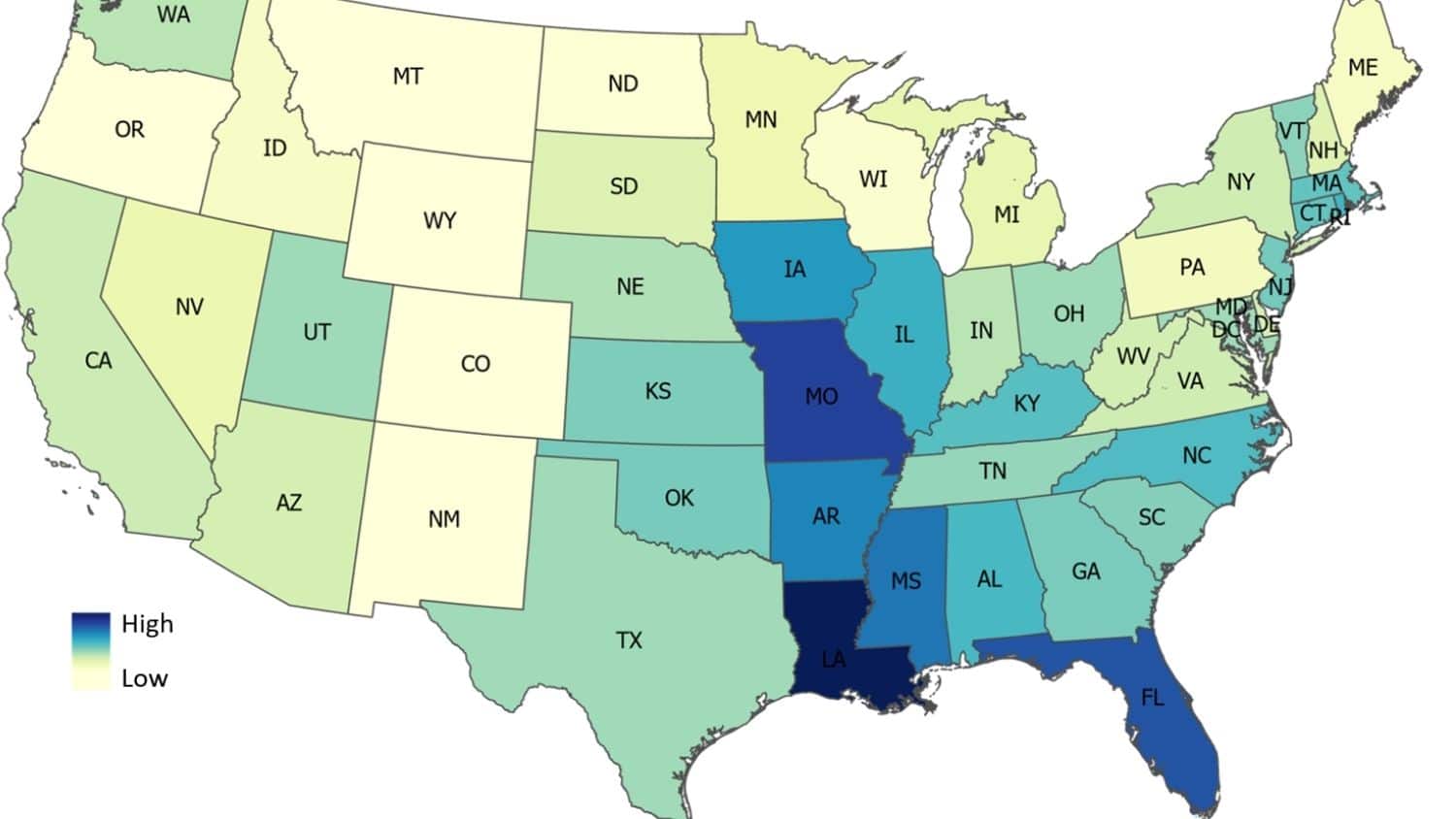
For Immediate Release
In a new study, North Carolina State University researchers used artificial intelligence to predict where flood damage is likely to happen in the continental United States, suggesting that recent flood maps from the Federal Emergency Management Agency do not capture the full extent of flood risk.
In the study, published in Environmental Research Letters, researchers found a high probability of flood damage – including monetary damage, human injury and loss of life – for more than a million square miles of land across the United States across a 14-year period. That was more than 790,000 square miles greater than flood risk zones identified by FEMA’s maps.
“We’re seeing that there’s a lot of flood damage being reported outside of the 100-year floodplain,” said the study’s lead author Elyssa Collins, a doctoral candidate in the NC State Center for Geospatial Analytics. “There are a lot of places that are susceptible to flooding, and because they’re outside the floodplain, that means they do not have to abide by insurance, building code and land-use requirements that could help protect people and property.”
It can cost FEMA as much as $11.8 billion to create national Flood Insurance Rate Maps, which show whether an area has at least a 1% chance of flooding in a year, according to a 2020 report from the Association of State Floodplain Managers. Researchers say their method of using machine learning tools to estimate flood risk offers a way of rapidly updating flood maps as conditions change or more information becomes available.
“This is the first spatially complete map of flood damage probability for the United States; wall-to-wall information that can be used to learn more about flood risk in vulnerable, underrepresented communities,” said Ross Meentemeyer, Goodnight Distinguished Professor of Geospatial Analytics at NC State.
To create their computer models, researchers used reported data of flood damage for the United States, along with other information such as whether land is close to a river or stream, type of land cover, soil type and precipitation. The computer was able to “learn” from actual reports of damage to predict areas of high flood damage likelihood for each pixel of mapped land. They created separate models for each watershed in the United States.
“Our models are not based in physics or the mechanics of how water flows; we’re using machine learning methods to create predictions,” Collins said. “We developed models that relate predictors – variables related to flood damage such as extreme precipitation, topography, the relation of your home to a river – to a data set of flood damage reports from the National Oceanic and Atmospheric Administration. It’s very fast – our models for the U.S. watersheds ran on an average of five hours.”
The actual flood damage reports they used to “train” the models were publicly available reports from NOAA made between December 2006 and May of 2020. Compared with recent FEMA maps downloaded in 2020, 84.5% of the damage reports they evaluated were not within the agency’s high-risk flood areas. The majority, at 68.3%, were located outside of the high-risk floodplain, while 16.2% were in locations unmapped by FEMA.
When they ran their computer models to determine flood damage risk, they found a high probability of flood damage for more than 1.01 million square miles across the United States, while the mapped area in FEMA’s 100-year flood plain is about 221,000 square miles. Researchers said there are factors that could help explain why the differences were so large, including that their machine-learning-based model assessed damage from floods of any frequency, while FEMA only includes flooding that would occur from storms that have a 1% chance of happening in any given year.
“Potentially, FEMA is underestimating flood damage exposure,” Collins said.
One of the biggest drivers of flood damage risk was proximity to a stream, along with elevation and the average amount of extreme precipitation per year. The three Census regions with the highest probability were in the Southeast. Louisiana, Missouri, the District of Columbia, Florida and Mississippi had the highest risk of any U.S. state or district in the continental United States. Of the 30 most high-risk counties, North Carolina had three: Dare, Hyde and Tyrrell.
In their model, researchers used historical climate data. In the future, they plan to account for climate change.
In the meantime, researchers say their findings, which will be publicly accessible, could be useful for helping policymakers involved in land-use planning. They also represent a proof-of-concept method for efficiently updating flood maps in the future.
“There is still work to be done to make this model more dynamic,” Collins said. “But it’s part of a shift in thinking about how we approach these problems in a more cost-effective and computationally efficient manner. Inevitably, with climate change, we’re going to have to update these maps and models as events occur. It would be helpful to have future estimates that we can use to prepare for whatever is to come.”
The study, “Predicting flood damage probability across the conterminous United States,” was published online Feb. 21, 2022, in Environmental Research Letters. In addition to Collins and Meentemeyer, other authors included Georgina M. Sanchez, Adam Terando, Charles C. Stillwell, Helena Mitasova and Antonia Sebastian. This project was supported by the U.S. Geological Survey Southeast Climate Adaptation Science Center (G19AC00083) and the North Carolina State University Sea Grant program (R/MG-2011).
-oleniacz-
Note to authors: The abstract follows.
“Predicting flood damage probability across the conterminous United States”
Authors: Elyssa L. Collins, Georgina M. Sanchez, Adam Terando, Charles C. Stillwell, Helena Mitasova, Antonia Sebastian and Ross K. Meentemeyer.
Published online in Environmental Research Letters on Feb. 21, 2022
DOI: 10.1088/1748-9326/ac4f0f
Abstract: Floods are the leading cause of natural disaster damages in the United States, with billions of dollars incurred every year in the form of government payouts, property damages, and agricultural losses. The Federal Emergency Management Agency oversees the delineation of floodplains to mitigate damages, but disparities exist between locations designated as high risk and where flood damages occur due to land use and climate changes and incomplete floodplain mapping. We harnessed publicly available geospatial datasets and random forest algorithms to analyze the spatial distribution and underlying drivers of flood damage probability caused by excessive rainfall and overflowing water bodies across the conterminous United States. From this, we produced the first spatially complete map of flood damage probability for the nation, along with spatially explicit standard errors for four selected cities. We trained models using the locations of historical reported flood damage events (n = 71,434) and a suite of geospatial predictors (e.g., flood severity, climate, socio-economic exposure, topographic variables, soil properties, and hydrologic characteristics). We developed independent models for each hydrologic unit code level 2 watershed and generated a flood damage probability for each 100-m pixel. Our model classified damage or no damage with an average area under the curve accuracy of 0.75; however, model performance varied by environmental conditions, with certain land cover classes (e.g., forest) resulting in higher error rates than others (e.g., wetlands). Our results identified flood damage probability hotspots across multiple spatial and regional scales, with high probabilities common in both inland and coastal regions. The highest flood damage probabilities tended to be in areas of low elevation, in close proximity to streams, with extreme precipitation, and with high urban road density. Given rapid environmental changes, our study demonstrates an efficient approach for updating flood damage probability estimates across the nation.
- Categories: