Researchers Use Geospatial Analytics and AI to Develop Global Food Security Solutions
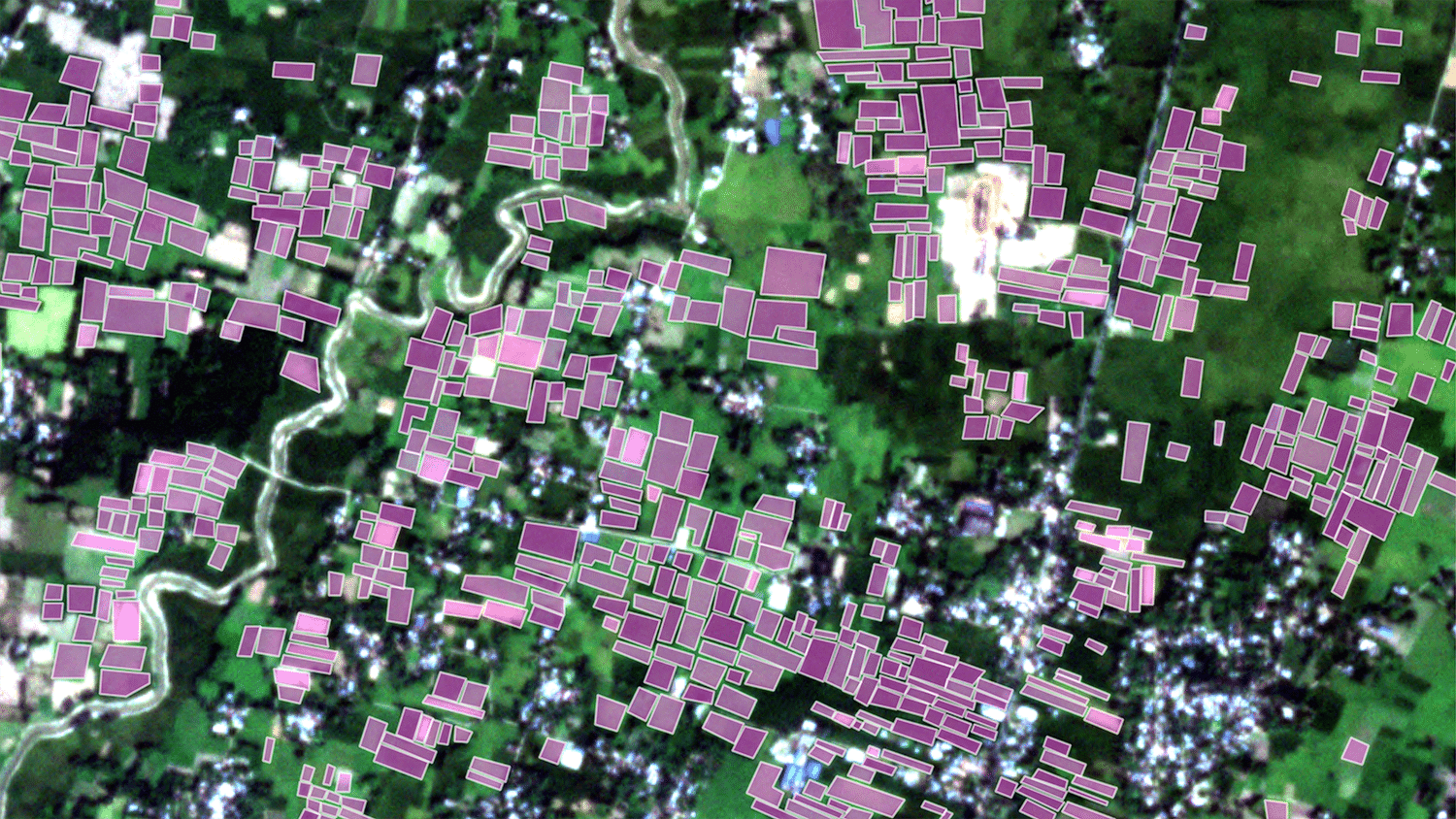
Climate change is forcing food producers to alter their processes in order to adapt to increasingly unpredictable and dangerous weather. Small farmers are especially vulnerable to the impacts of climate change, as they often lack the resources needed to pivot their crops and growing techniques. At NC State, researchers are integrating AI into geospatial technology to help solve these complex challenges to global food security and poverty.
Josh Gray is an associate professor in the College of Natural Resources who works with the Department of Forestry and Environmental Resources and with the Center for Geospatial Analytics. Remote sensing uses satellites to examine Earth’s physical characteristics from afar.
“If you want to measure the same thing everywhere, wall to wall, and you want to do it every day, remote sensing’s got you covered,” Gray said. “There are no other technologies that allow you to do that. It’s a really important scaling technology.”
There are no other technologies that allow you to do that.
“We can make measurements from orbit that we can’t make on the ground,” Gray added. “It doesn’t matter how many graduate students you can send out to the field — you just can’t measure things like how much water there is half a kilometer under the ground.”
At NC State’s annual University Research Symposium, Gray described how his lab uses geospatial technology and machine learning to study small farms in the Indo-Gangetic Plain and to identify solutions to problems they face on a warming planet.
Smallholders are those who own very small farms. Together, they produce more than half of the calories consumed by humans across the world. Along the Himalayas, these smallholders are disproportionately impoverished and affected by climate change.
These smallholders typically grow rice and wheat. Wheat is especially sensitive to increasing heat. To determine whether planting wheat earlier in the season could protect the crop from rising temperatures, Gray’s lab developed a process for determining planting dates across these small farms from series of satellite images.
These farms are less than 2 hectares each — or about 5 acres — and there are tens of millions of them in the region. It’s difficult to manually trace the boundaries of each farm. The lab trained a convolutional neural network to automatically identify farms via satellite images.
First, the lab used data augmentation to create image samples. These samples were based on existing images but had been flipped horizontally or vertically, rotated at different angles, blurred or had different levels of brightness.
These image samples were input into U-Net, a convolutional neural network architecture. Known as CNNs, convolutional neural networks are computational models in machine learning that can analyze visuals. Trained by these image samples, the U-Net could identify precise field boundaries.
This project, Gray said, is “a blend of conventional statistics, domain knowledge and then AI and machine learning sprinkled in.” Combining these disciplines creates a more capable and dynamic research process. “Knowing what time periods to select the imagery from allowed us to have a much more efficient machine learning pipeline,” Gray said.
Gray’s lab plans to scale the process in order to analyze the millions of farms in the region. With this research, the team could determine feasible solutions for smallholder farmers and bolster food security.