Following the Path: Making Huge Calculations Predictable
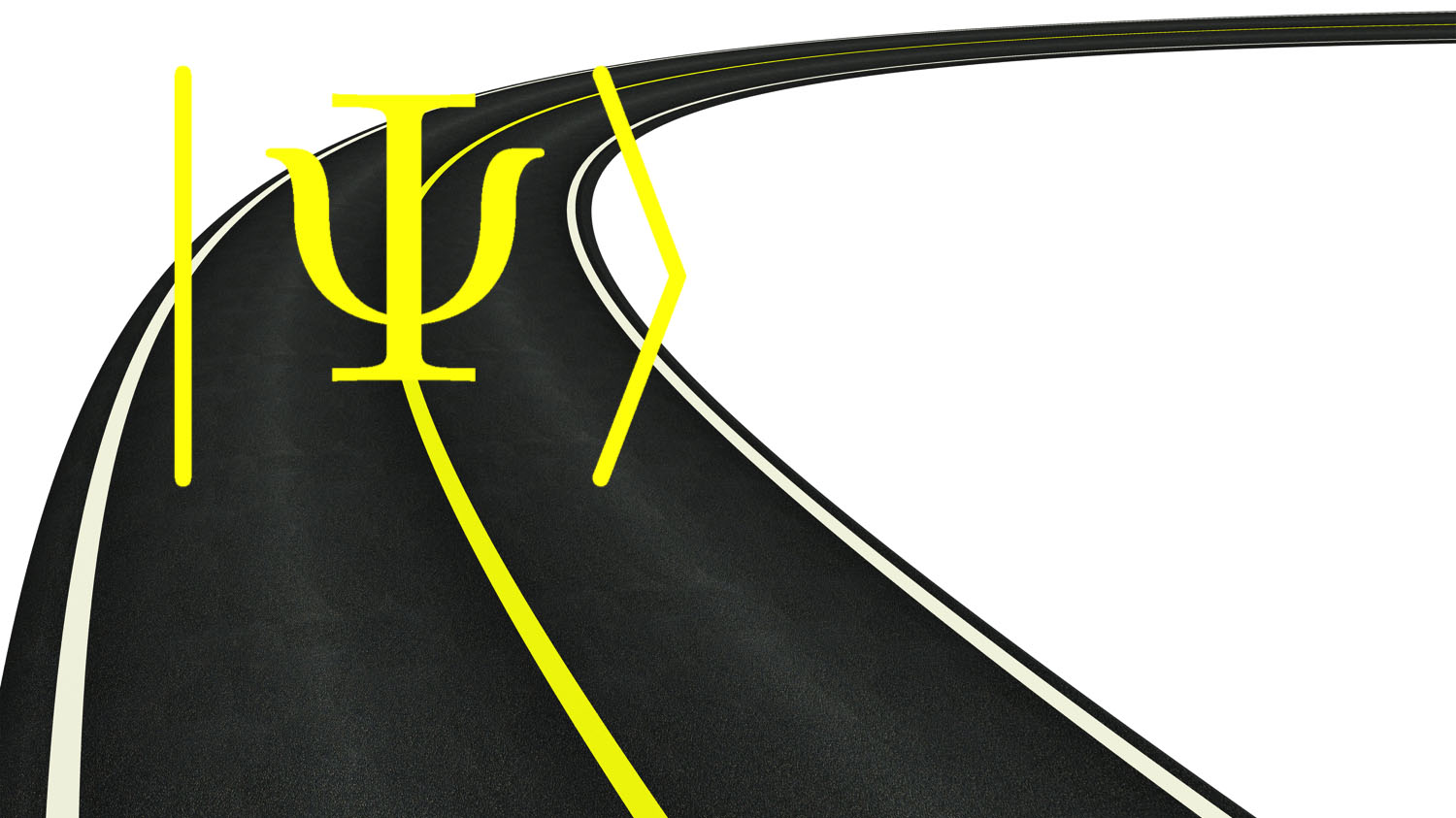
A team that includes nuclear physicists, a machine-learning researcher and an NC State mathematician has created a new approach for narrowing down huge calculations – such as those involved in quantum physics – by focusing on a single, predictive parameter. This approach, dubbed the eigenvector continuation, could prove useful in solving other scientific and engineering problems where the calculations are simply too large for computer memory to handle.
Dean Lee, former NC State physicist currently at Michigan State University, studies quantum particles and their interactions. “In quantum physics, matter has properties associated with waves, so looking at the wave function of a system tells us about its quantum structure,” Lee explains. “We are really interested in knowing the wave function of a system, especially when the system has cooled down to some low-energy state. But this is a very difficult problem. The amount of information packed into the wave function is often so large that even representing a single wave function in computer memory might be impossible.”
Lee was interested in finding a way to narrow down the amount of information a computer would need to process without affecting the accuracy of the calculations. He enlisted the help of NC State mathematician Ilse Ipsen to do so.
“Even though the wave function is very complicated, it is nevertheless completely determined by a few simple features like the particle interactions governing the system,” Lee says. “So we focused on one parameter within the system and looked at how the wave function changed as we varied that parameter. In this case, we chose the parameter that controls the strength of the particle interactions within the system.”
The team made small changes to the parameter they chose, then observed the wave function. “We saw that the wave function didn’t zig and zag in a bunch of different random directions; instead it bent smoothly with notable displacements in only a few directions,” Lee says. “The smooth variation means that we can predict what the wave function will be even in cases where the problem is otherwise impossible to solve.”
“It’s like viewing a series of roads or paths from atop a mountain, and then picking the route that has all the properties you need,” Ipsen says. “The computation is focused on the small space where all the interesting stuff happens, and you can extrapolate from there to find answers about the system as a whole.
“We call this approach the eigenvector continuation – you find the path and continue along it in small steps. The eigenvector is the property of the system that you’re looking at. It’s a way to narrow down huge computations to a smooth predictable computation while keeping errors at bay.”
Ipsen and Lee believe that the method also holds promise for similar “too big to handle” computations in other scientific or engineering fields. Their research appears in Physical Review Letters. Lee is corresponding author. Michigan State graduate students Dillon Frame and Rongzheng He, Cornell Tech and Samsung machine learning theorist Daniel Lee, formerly at the University of Pennsylvania, and Ermal Rrapaj, physicist from the University of Guelph, Ontario, contributed to the work.
- Categories: