How a ‘Digital Twin’ Can Make Wireless Networks Faster, More Reliable
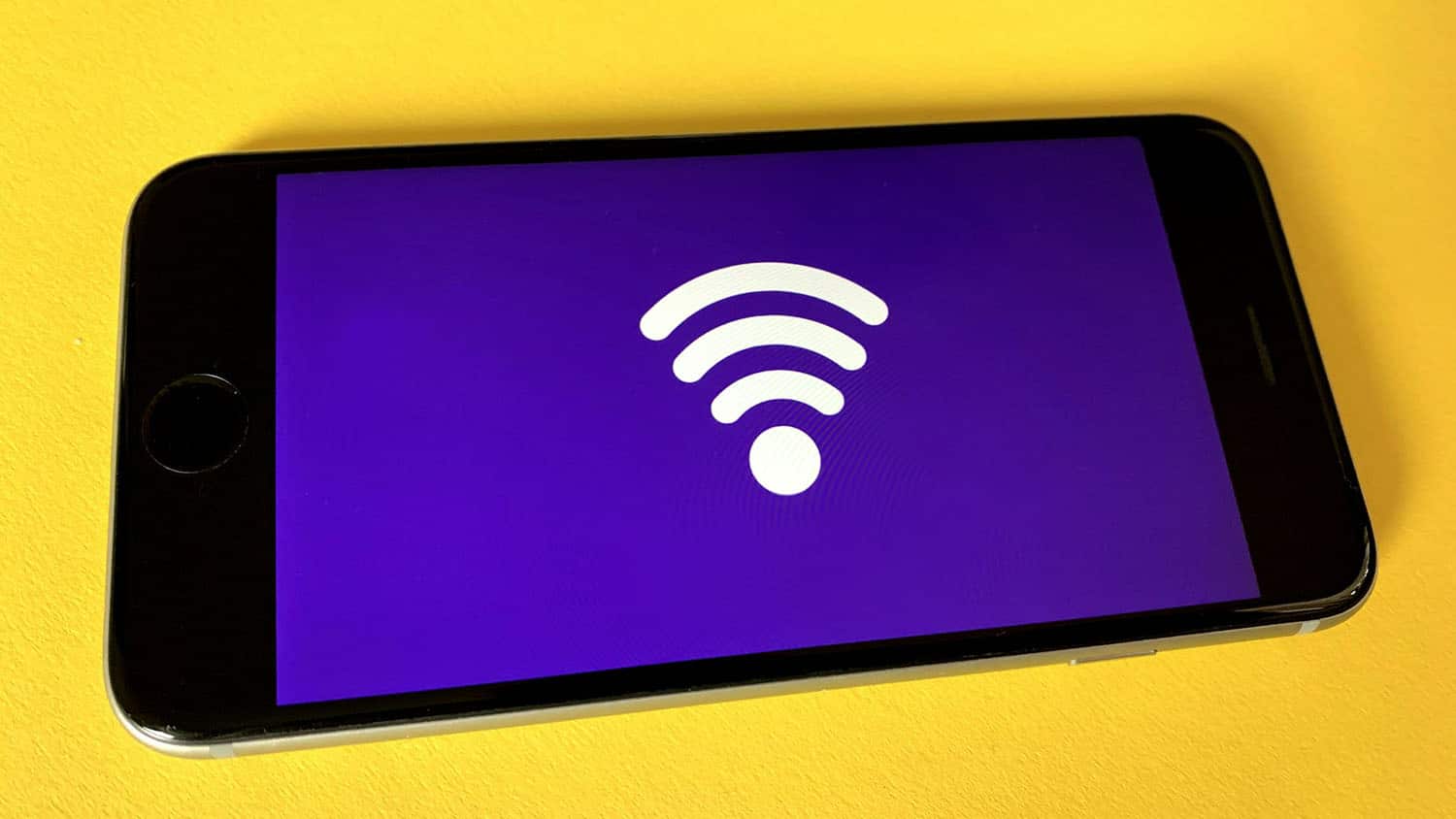
For Immediate Release
Computer science researchers have developed a new method for predicting what data wireless computing users will need before they need it, making wireless networks faster and more reliable. The new method makes use of a technique called a “digital twin,” which effectively clones the network it is supporting.
At issue is something called edge caching. Caching refers to storing data on a server that a system or network thinks users will be using (or re-using) in the near future. This allows the system to meet user demands more quickly than if the system had to retrieve the data from the original source. Edge caching is when a system is caching data in the server that is closest to the end user, such as computers that are incorporated into network routers or colocated with those routers.
“Two big challenges here are determining which data need to be cached and how much data the edge server should store at any given point in time,” says Yuchen Liu, corresponding author of a paper on the work and an assistant professor of computer science at North Carolina State University. “Systems can’t put everything in edge caches, and storing too much redundant data on an edge server can slow down the server if the data are using too many computational resources. As a result, systems are constantly making decisions about which data packages to store and which data packages can be evicted.
“The more accurate a system is at predicting which data users will actually want, and how much data the edge servers should be storing, the better the system’s performance,” Liu says. “Our work here focused on improving those predictions.”
The new edge caching optimization method, called D-REC, makes use of a computational modeling technique called a digital twin. A digital twin is a virtual model of a real object. In the case of D-REC, the digital twin is a virtual model of a defined wireless network – whether that’s a cellular network or a Wi-Fi network.
“The method can be applied to any wireless network, depending on the system administrator or network operator’s needs,” says Liu. “D-REC can be adjusted depending on the needs of the user.”
In D-REC, the digital twin takes real-time data from the wireless network and uses it to conduct simulations to predict which data are most likely to be requested by users. These predictions are then sent back to the network to inform the network’s edge caching decisions. Because the simulations are performed by a computer that is outside of the network, this does not slow down network performance.
The researchers used open-source datasets to determine whether a wireless network operated more efficiently with D-REC. The researchers ran extensive experiments designed to account for many variables, such as the scale of the network, the number of users on a network, and so on.
“D-REC outperformed conventional approaches,” says Liu. “Our technique improved the network’s ability to accurately predict which data should be edge cached. D-REC also helped systems do a better job of balancing data storage across their networks.”
In addition, because D-REC’s digital twin focuses on predicting network behavior, it can identify potential problems in advance.
“For example, if the digital twin thinks there is a high likelihood that a specific base station, or server, will be overloaded, the network can be notified – allowing it to redistribute data across the network in order to preserve network performance and reliability,” says Liu.
“At this point, we’re open to working with network operators to explore how D-REC can improve network performance and reliability in real-world situations.”
The paper, “Digital Twin-Assisted Data-Driven Optimization for Reliable Edge Caching in Wireless Networks,” is published in the IEEE Journal on Selected Areas in Communications. First author of the paper is Zifan Zhang, a Ph.D. student at NC State. The paper was co-authored by Zhiyuan Peng, a postdoctoral researcher at NC State; Dongkuan Xu, an assistant professor of computer science at NC State; Mingzhe Chen of the University of Miami; and Shuguang Cui of the Chinese University of Hong Kong.
This work was done with support from the National Science Foundation under grants 2312138, 2312139 and 2332834.
-shipman-
Note to Editors: The study abstract follows.
“Digital Twin-Assisted Data-Driven Optimization for Reliable Edge Caching in Wireless Networks”
Authors: Zifan Zhang, Yuchen Liu, Zhiyuan Peng, and Dongkuan Xu, North Carolina State University; Mingzhe Chen, University of Miami; and Shuguang Cui, The Chinese University of Hong Kong
Published: July 22, IEEE Journal on Selected Areas in Communications
DOI: 10.1109/JSAC.2024.3431575
Abstract: Optimizing edge caching is crucial for the advancement of next-generation (nextG) wireless networks, ensuring high-speed and low-latency services for mobile users. Existing data-driven optimization approaches often lack awareness of the distribution of random data variables and focus solely on optimizing cache hit rates, neglecting potential reliability concerns, such as base station overload and unbalanced cache issues. This oversight can result in system crashes and degraded user experience. To bridge this gap, we introduce a novel digital twin-assisted optimization framework, called D-REC, which integrates reinforcement learning (RL) with diverse intervention modules to ensure reliable caching in nextG wireless networks. We first develop a joint vertical and horizontal twinning approach to efficiently create network digital twins, which are then employed by D-REC as RL optimizers and safeguards, providing ample datasets for training and predictive evaluation of our cache replacement policy. By incorporating reliability modules into a constrained Markov decision process, D-REC can adaptively adjust actions, rewards, and states to comply with advantageous constraints, minimizing the risk of network failures. Theoretical analysis demonstrates comparable convergence rates between D-REC and vanilla data-driven methods without compromising caching performance. Extensive experiments validate that D-REC outperforms conventional approaches in cache hit rate and load balancing while effectively enforcing predetermined reliability intervention modules.